DEPARTMENTS
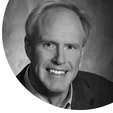
RISK
JOHN MULHAUSEN, PhD, CIH, CSP, FAIHA, retired in 2018 from 3M where he worked for 31 years in a variety of global health and safety risk management roles, most recently as director of corporate safety and industrial hygiene.
Send feedback to The Synergist.
One Is the Loneliest Number
Addressing Our Low-n Conundrum Using Modern Bayesian Statistical Tools
BY JOHN MULHAUSEN
When defining a sampling strategy to characterize a similar exposure group’s (SEG’s) exposure profile, limiting the strategy to only one sample is not a good idea for a whole host of reasons. We are understandably uncomfortable making exposure risk decisions when we have very few opportunities to measure exposures. We’d love to have six or 10 samples—the more, the better—each time we make an exposure risk decision. But reality often forces us to make decisions with far fewer measurements. It is likely that each of us has faced a situation where, due to practical, operational, or even temporal constraints, we feel fortunate to have obtained one sample and must now make a decision based on that single result. How can we ensure that our decision is as good as it can be?
The good news is that the modern Bayesian statistical tools freely available from AIHA are ready, willing, and able to provide scientific analysis to help us make the exposure risk decision and communicate the results—even with low numbers of samples, including just one. The additional good news is that, with careful planning, we often have opportunities to expand the number of samples and reduce the uncertainty in the decision, if needed. The results of the statistical analysis help us determine priorities for that additional follow-up.
A TALE OF THREE SEGS
Bayesian tools for interpreting exposure data take advantage of prior knowledge regarding likely workplace exposure variability. They use that knowledge to expand upon the information provided by very small numbers of samples to help us make accurate exposure risk decisions. So even though our single sample result tells us nothing about the exposure variability in the measured SEG, if we are comfortable with the Bayesian tool’s assumptions about lognormality and possible variability for our SEG, we can take advantage of that prior knowledge. That is why AIHA’s free training, “Improving Exposure Judgments: An Introduction to IH Statistics,” places such an emphasis on using the statistical tools every time we have monitoring data. Doing so helps us learn about the variability extant in our workplace SEGs so that we can be comfortable with, or change, the tool’s assumptions.
Regrettably, use of free statistical tools is quite low, and many OEHS professionals seem misinformed about them.
Of course, the adage “garbage in, garbage out” certainly applies to our statistical tools, which rely on the assumption that the data are scientifically collected in a manner that represents the underlying exposure profile of the SEG. Many variables can bias results if they’re unaccounted for in the sampling strategy and data interpretation. These variables include the worker, the work shift, process or task inconsistency, unobserved quality control issues with the sample or analysis, and the time of year when samples are collected. Because our statistical tools cannot account for these potential errors, we must be aware of these issues when making exposure risk decisions even with large sample sizes. When our dataset is very small, we must be hypervigilant.
Figure 1 illustrates how Bayesian statistical tools help us make exposure risk decisions, develop prioritized follow-up plans, and communicate the results for three different SEGs with a single sample result. The Bayesian decision analysis (BDA) results depicted in Figure 1 were generated by Expostats, a tool that estimates risk and uncertainty based on measurement data entered by the user. The bar graphs refer to the exposure categories defined in the AIHA publication A Strategy for Assessing and Managing Occupational Exposures, which are used to characterize the acceptability of exposures and guide appropriate follow-up exposure management. These categories range from “no action needed” (category 0) to “unacceptable” (category 4).
Click or tap on the figure to open a larger version in your browser.
The 95th percentile exposure for SEG A is likely less than 10 percent of the 100 ppm occupational exposure limit and therefore in category 1 as represented by the highest bar with a probability of 67.1 percent. The BDA chart also indicates only a 4.8 percent chance that the 95th percentile exceeds the OEL (category 4), and therefore a greater than 95 percent probability that the 95th percentile exposure is less than the OEL. In accordance with guidance in the “Principles of Good Practice for the Industrial Hygienist/Occupational Hygienist,” the SEG exposure is deemed acceptable with low priority for additional follow-up, particularly as compared with SEGs B and C.
The single sample result of 20 ppm for SEG B is close enough to the OEL of 100 ppm that the potential uncertainty is too great to make a distinction as to whether the 95th percentile exposure is in category 2 (36.3 percent likely), category 3 (24 percent likely), or category 4 (38.7 percent likely). Due to the high uncertainty and the substantial likelihood that the exposure could be category 3 or 4 (62.7 percent), the exposure is deemed uncertain/unacceptable and given a high priority for further monitoring with consideration given to implementing short-term controls, such as respirator use, until the monitoring results can be collected and analyzed to resolve the uncertainty.
In the case of SEG C, the Bayesian statistical analysis indicates that the single result of 70 ppm is close enough to the OEL that its 95th percentile has an 85.9 percent likelihood of being in category 4 regardless of whether the exposure profile has high or low variability. Exposures would be deemed unacceptable with high priority given to implementing controls. In actual practice, these initial controls might be for the short term while additional monitoring is performed to better understand the exposure profile and facilitate the development of more robust solutions further up the hierarchy of controls.
STRENGTHENING OUR PRACTICE
These examples show how, even when sample sizes are very small, the Bayesian decision analysis charts provide a consistent framework for determining exposure risk and defining and prioritizing follow-up activities. They are a straightforward mechanism for communicating risks, priorities, and follow-up activities to stakeholders.
Regrettably, however, use of Expostats and other free statistical tools is quite low, and many OEHS professionals seem misinformed about them. In a 2023 survey of OEHS professionals, 70 percent of 340 respondents indicated that the reason they don’t conduct statistical analysis is because they “do not usually have enough monitoring data.” This excuse is nonsensical, as demonstrated by the examples in this article. Using statistical tools every time we have monitoring data will strengthen our practice and improve the effectiveness and efficiency of our exposure risk management programs.
RESOURCES
AIHA: A Strategy for Assessing and Managing Occupational Exposures, 4th ed. (2015).
AIHA: “AIHA Risk Assessment Tools.”
AIHA: “Airborne Chemical Exposure Assessment Survey” (PDF, June 2023).
AIHA Guideline Foundation: “Principles of Good Practice for the Industrial Hygienist/Occupational Hygienist.”
AIHA: “Improving Exposure Judgments: An Introduction to IH Statistics.”
AIHA: “Improving Exposure Judgments (IEJ).”
AIHA: “Making Accurate Exposure Risk Decisions.”
The Synergist: “Filling the Gaps: Survey Clarifies Needs for Improvement in Exposure Assessment” (January 2024).