DEPARTMENTS
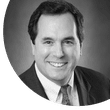
ETHICS
ALAN LEIBOWITZ, CIH, CSP, FAIHA, is the president of EHS Systems Solutions LLC, chair of the Joint Industrial Hygiene Ethics Education Committee, past chair of the Board for Global EHS Credentialing, and a past Board member of AIHA.
Send feedback to The Synergist.
AI in OEHS: Ethics of the Future
BY ALAN LEIBOWITZ
Editor’s note: The case study in this article is fictitious and is intended to highlight ethical issues in the practice of industrial hygiene. Any resemblance to real people or organizations is coincidental. The opinions expressed in this article are those of the author and do not necessarily reflect the opinions of AIHA, The Synergist, the JIHEEC, or its members.
Please share your thoughts on this article by emailing the Synergist team or submitting them through the form at the bottom of this page. Responses may be printed in a future issue as space permits.
Some information in this article resulted from a prompt to online chatbots and is included here for illustrative purposes. The Synergist does not accept submissions generated by artificial intelligence tools.
Artificial Intelligence (AI) is the new “big thing” despite having been in development since the dawn of computing. As a field of study, AI’s reported roots are often traced to the “Dartmouth Summer Research Project on Artificial Intelligence” in 1956. In the last few decades, AI has been increasingly incorporated, behind the scenes, into many systems in fields such as technology, entertainment, and finance. What has changed is computing power and the creation of tools that provide easy access to sophisticated generative AI language models whose main function is to produce content. These AI models are trained on massive datasets generated by scraping various sources, which are used in iterative cycles of model improvement. The AI can then predict which word follows the next in any given sentence. At the current state of AI evolution, this ability allows tools such as ChatGPT, Bard, and Bing AI to write plausible-sounding statements that are often surprisingly accurate.
Users of AI tools powered by large language models (LLMs) can ask natural-language questions in a broad range of subject areas. Refining those questions for optimal content creation has led to a new specialty, AI prompt engineering. However, even with optimized prompts, responses cannot be accepted at face value. These systems do not inherently possess “knowledge” or “facts” in the way humans understand them. An LLM-based tool is optimized to provide the most likely response—not the most correct one—based on its training data. For example, if an LLM was trained mainly on data related to a specific type of hazard (chemical hazards, for example) it might overemphasize that concern and downplay or ignore others (such as biological or physical hazards). Responses are only as good as the datasets they have been trained on.
An OEHS example of errant responses can be seen from a recent prompt that asked an LLM to create a timeline of asbestos regulation. The response cited an impressive-sounding paper—“Gilson, J. C., & Sullivan, M. C. (1960). Asbestosis: A Review of the Literature, British Journal of Industrial Medicine, 17(4), 260-271”—that unfortunately did not exist. Instead, a landmark paper by C. Wagner, C. A. Sleggs, and P. Marchand regarding mesothelioma appears in that journal, on those same pages, in that same year. While an incorrect citation is easy to identify, unwarranted user confidence in LLM output has already had real-world consequences. Lawsuits have been filed relating to allegedly fabricated results, and it would be easy to imagine the consequences of trusting AI to create an emergency response plan or a safety data sheet without appropriate technical review.
While technical concerns exist with the current iterations of AI, they will likely diminish over time as the systems become more sophisticated and are trained on larger datasets. This increasing capability will not eliminate all risk or the ethical challenges that AI can introduce. As OEHS professionals begin to incorporate AI assistance in developing and managing their programs, some ethical elements should be considered, including the following, which were identified with the help of ChatGPT and Bard:
Data privacy. Personal and sensitive information will be collected from workers. Ensure that informed consent is documented and the data is protected from unauthorized access, misuse, or breaches. Data collection, storage, and processing must adhere to privacy laws and regulations.
Bias and fairness. AI algorithms can perpetuate biases present in the data they are trained on. Biased AI systems could result in unequal treatment or protection for certain groups of workers. Users must carefully curate and review training data to minimize bias and ensure fairness in the algorithm’s decision-making processes. Regular monitoring and auditing of AI systems are necessary to identify and address any biases that may arise.
Accountability and transparency. OEHS professionals are responsible for the decisions made by AI systems that they incorporate into their practice. Use of AI should be noted, and all facts and recommendations must be validated. Rigorous testing and validation processes should be in place to avoid potential risks or unintended consequences.
Equipment maintenance and calibration. AI systems in OEHS will often rely on real-time data from sensors and monitoring devices throughout a facility. If the operation neglects proper maintenance and calibration of these instruments, the result may be faulty readings and inaccurate inputs for the AI system. Consequently, hazardous conditions go undetected, putting employees at risk.
These systems do not inherently possess “knowledge” or “facts” in the way humans understand them.
Human supervision and decision-making. While AI can assist in decision-making processes, it should not replace human expertise and judgment entirely. Human supervision is essential to interpret AI-generated insights, consider contextual factors, and make final decisions. Maintaining a balance between AI automation and human intervention is necessary to ensure responsible and ethical use of AI.
Addressing these ethical concerns requires a multidisciplinary approach involving OEHS professionals, data scientists, ethicists, policymakers, and stakeholders. It is important to foster open dialogue, establish guidelines and standards, and promote ethical frameworks to guide the development, deployment, and use of AI in OEHS practices.
UNINTENDED CONSEQUENCES
Tobor was a young industrial hygienist working for one of the larger environmental consulting firms in the state. As the junior member of his team, he was often assigned some of the more tedious tasks in any given project. The new contract with Armored Man Inc., a global manufacturer of defense equipment, was no exception. Dozens of Tobor’s coworkers traveled around the world collecting data and auditing sites. He was assigned to summarize the collected information along with large volumes of historic corporate data to assist his project managers in making action plans for the client.
Tobor was aware of the usefulness of AI and had used it many times to help prepare reports. While the volume of data for this project was an order of magnitude greater than for his previous AI uses, Tobor had confidence in the system. He was also enthusiastic about the time he would save, which could be devoted to more interesting work. Tobor prompted the system to summarize the data with particular attention to applicable regulations and any exposure hazards that required action.
The output looked great, and, after a brief review, Tobor submitted it to his management without mentioning the use of AI. They were impressed with how quickly he provided the summary and based their recommendations to the client on the data he provided. Unfortunately, in discussions with the client, it became clear that some of the international standards identified in the data summary did not exist or were inaccurately applied to the issue being addressed.
FOR DISCUSSION
As Tobor looked for a new position, he asked himself some questions: How could he have better incorporated AI into his task? What obligations did he have to let the team know he was using AI to complete his assigned task? How could he have more effectively validated the data? Does AI have a place in OEHS practice?